In this short series, we identify keys to successfully integrating AI into your business. First, a look at the stage before project kick-off – defining objectives.
ChatGPT is a superb piece of software. If you haven’t tried it, you should. It’s free to sign up and test out. Similarly, DALL·E 2, Midjourney and Stable Diffusion can generate pretty lifelike images instantly given simple text prompts. You may have seen an example of the Pope wearing a puffer jacket doing the rounds over the weekend, an image generated by Midjourney (hint: look at the hands — artificial intelligence (AI) engines haven’t gotten them right yet).
It’s important in our sphere to keep track of these emerging technologies and to keep them in mind when considering our strategic objectives. But critically, they shouldn’t distract us from the core problems we’re trying to solve in our businesses or from the exciting opportunities we’re looking to exploit. AI is such a broad term, covering everything from voice assistants to machine learning models to self-driving car technology. It’s easy to be a little overwhelmed and not know where to start.
We should ask the question “What can AI do for us?” rather than “What can we do with AI?” The subtlety being that we first understand what we’re trying to resolve before we try to leverage solutions.
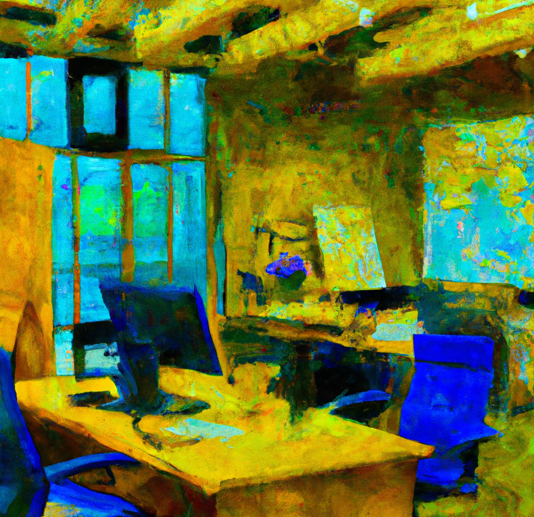
Define the objective
The most critical part of any AI initiative is to identify the problem you’re going to solve or opportunity you’re going to exploit. What are the pieces of information or tools that will help you to achieve your objectives?
A common misconception of AI is that you can collect a bunch of data and do data science on it to find patterns. This is only partially true. You will find patterns but without a defined problem, they’re likely to be entirely useless (e.g., people with an A in their name are more likely to get an even number payment amount) or entirely obvious to people with domain knowledge (claims on policies with indexation clauses increase in payment amount year-over-year).
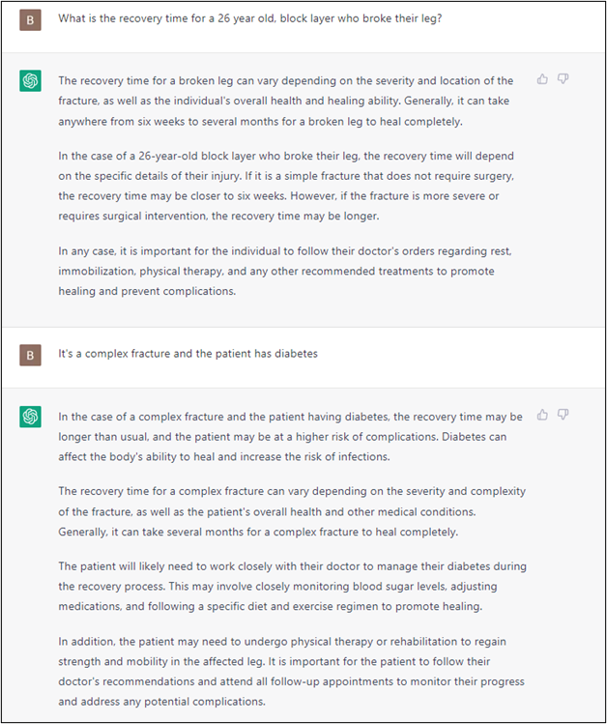
If you consider an example like predicting disability claim duration, you need to ask more questions. Is your primary objective to improve operational efficiency through automation or triage? Are you trying to predict total cost of claim for reserving? Do you want to give greater guidance to newer claims handlers? Or are you forecasting future staffing requirements? Potential claim duration is a useful information point for any of these objectives. But if you understand your primary objective, you can achieve more with the same data.
For triage, you’ll want to know the extent to which durations can be impacted by more active claim management. If the duration of a claim is highly predictable, it would not be valuable to assign expensive resources to it. On the other hand, if a claim shows a high variability in potential outcomes, interventions could yield a high return on investment.
For reserving, a straight average duration prediction may be best. For training, you might opt for a more specialized version of the ChatGPT example above. While it’s low on detail, it’s helpful for prompting the right next question. Future staffing requirements could incorporate all the above along with other nuances of duration.
The AI landscape is strewn with projects that failed to meet objectives that were not defined properly in the first place. Thankfully though, teams with a well-defined objective have a very good chance of succeeding once the right type data is available and integrating the solution into the business process is well understood. We’ll cover those topics in future blogs in this series.
A purpose-built solution
FINEOS works with our customers to identify common industry problems that may be solved by AI. We develop models for the life, accident, and health industry, delivering complete end-to-end solutions. We start with the business objectives and operationalize solutions. We work backwards through data identification and then forward through extraction and model development. The result is smart workflows empowered by seamless customer-specific AI.
Read more in our white paper: You Already Have What It Takes: Machine Learning for Group Insurers.